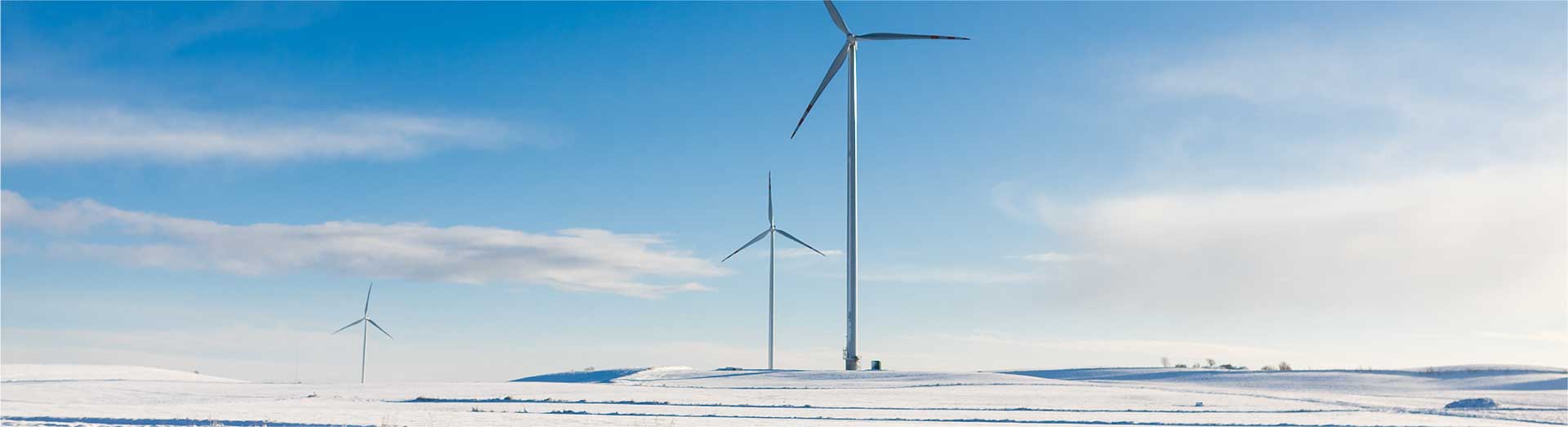
Intelligent Wind Turbines for Cold Climates
Funder
The Royal Society
Value
£11,975 value to Coventry University
Collaborators
Université Laval and Nergica
Project Team
(PI) Dr Jonathan Nixon; (Co-I) Prof. James Brusey;
(Co-Awardee) Prof. André Bégin-Drolet; Charles Godreau
Duration of Project
02/11/2022 - 01/05/2025
Project Overview
More than a quarter of the world’s wind turbines are installed in cold climates and suffer from icing, which damages turbines, causes dangerous break-off ice and reduces annual power generation by up to 20%. Wind energy investment is growing significantly in cold high-altitude regions with an abundance of wind energy. However, there are no commercially available or widely accepted solutions to address the icing problem, which costs the industry hundreds of millions of pounds every year.
Wind turbine installations in Canada and Scotland experience a range of different icing conditions and they are particularly compromised by the prevalence of icing. The most common outcome for operators is to see wind turbines shut down when too much ice has formed on the blades. The best operational decision for a wind turbine during a cold weather event is a highly complex problem and depends on the type, duration and frequency of an icing event.
For the first time, an icing sensor – developed by Université Laval and commercialised by Instrumentation Icetek – has now been installed across a number of wind farms in Canada, which can make operators aware of ice build-up and ice type. The aim now is to demonstrate that intelligent wind turbine decision-making algorithms could be applied by operators to effectively reduce the icing problem, thus enabling wider global use of wind energy and lowering energy costs for consumers.
Project Objectives
- Engage wind turbine manufactures/operators and visit wind farms sites in Canada and UK.
- Prepare wind farm cold climate and ice sensor datasets and evaluate alternative predictive AI methods for ice detection.
- Model annual output for wind turbines under different AI-informed cold climate control strategies.
- Demonstrate advancements made in cold climate AI models to different wind turbine stakeholders.