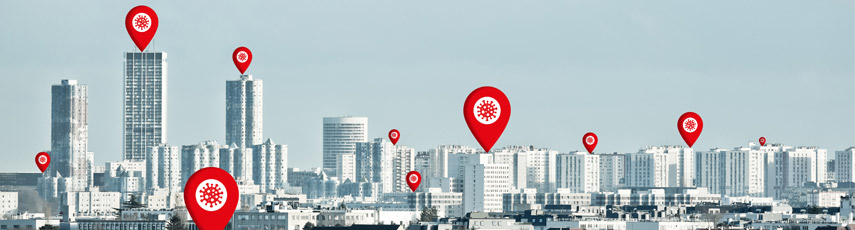
Compressive Population Health: Cost-Effective Profiling of Prevalence for Multi Non-Communicable Diseases via health Data Science
Funder
EPSRC
Team
Collaborators
Prof Sumi Helal- Lancaster University
Prof Alf Collins- NHS England
Dr Duncan Vernon- Warwickshire County Council
Duration
1 October 2021 to 30 September 2023
Value
£223,118.96
Project overview
With a growing ageing population and changes in lifestyles, non-communicable diseases (NCD), e.g. heart disease, diabetes, and cancer, have become extremely prevalent in our society, and the situation is more challenging in UK compared to other developed countries. Population health monitoring is a fundamental block for public health services, and profiling population-scale prevalence of multiple NCD across different regions (e.g., building the spatially fine-grained morbidity rate map) is one of the most important tasks. However, traditional public health data collection and prevalence profiling approaches, such as clinic-visit-based data integration and health surveys, are often very costly and timeconsuming.
This project proposes a novel paradigm, called compressive population health (CPH for short), to reduce the data collection cost during the profiling of prevalence to the maximum extent.
The basic idea CPH is that a subset of areas is intelligently selected for data collection and population health profiling in the traditional way, while leveraging inherent data correlations to perform data inference for the rest of the areas. CPH is facilitated by the exploitation of the following types of inherent data correlations found by epidemiologists. (a) Intra-Disease Spatial Correlations. That is, regions are more similar in the prevalence rate of some diseases when they are neighbouring, or share certain common environmental, socioeconomic, and demographical attributes. (b) Inter-Disease Correlations. Multimorbidity, commonly defined as the co-presence of two or more chronic conditions, demonstrates that statistics for different types of disease may also correlate with each other. For example, regions with higher obesity rate are more likely to have higher rates of heart disease and cancers.
The proposed CPH is a novel solution to a public health data collection challenge enabled by data science and artificial intelligence. It opens the door for a disruptive population health monitoring paradigm with potential significant cost reductions for public health authorities. By closely working with partners from public health sector, including NHS England and Public Health at Warwickshire County Council, we will evaluate the feasibility of this approach based on multiple public health datasets together with relevant demographic/geographic statistics in the same regions.
Project objectives
Profiling population-scale prevalence of non-communicable diseases (NCD) across different regions is crucial for a nation's public health surveillance system, which helps decision makers, health planning administrators, pharmaceutical manufacturers, and clinicians, to effectively treat disease, allocate medical resources, and manage population health. However, traditional public health data collection and prevalence profiling approaches, such as clinic-visit-based data integration and health surveys, are often very costly. To tackle this urgent challenge, this project aims to propose a novel health data science paradigm called Compressive Population Health (CPH) that aims to reduce the cost to the maximum extent for the profiling of prevalence rate of multiple NCD. In addition to the major goal of cost reduction, we should also make sure that the obtained prevalence profiles are reliable. The expected transformative outcome of this project is to benefit the public health authorities (e.g., NHS and Public Health England) in reducing the economic burden on the population health surveillance tasks
Our basic vision is that, for each target disease (e.g., obesity, hypertension, and diabetes), CPH only selects its "best" subset of regions (called Traditionally-Sensed Areas, TS-A for short) where public health administrators still profile the prevalence rate through traditional method (either by hospital-visit-based data integration or survey-based approach). Then, CPH uses prevalence rate measured from TS-A to perform inference on the un-selected regions (called "Inferred Areas", IF-A for short). This inference is facilitated by exploiting both the Intra-disease spatial correlations and inter-disease correlations extracted from historical data in multiple open-access public health datasets and exiting evidences from epidemiology research communities. In order to realize this idea, this project develops three technical work packages to accomplish the following technical goals: (1) Investigate and extract latent data correlations and further utilize them to build learning models for prevalence inference on the target geographical grids. (2) Design intelligent algorithms for selecting TS-A for each disease with multi-objective optimization goals including cost, reliability, and latency. (3) Evaluate and interpret the inference results of prevalence rate in IF-A to ensure the reliability and robustness of the approach.
Impact statement
The following stakeholders will benefit from the deliverables or the long-term impact of this project.
- Government and public health authorities: Profiling population-scale prevalence of different NCD across different regions is an important task in the public health surveillance system. Traditional approaches, such as clinic-visit-based data integration and health surveys, are often very costly and time-consuming. As a nation we're living longer than ever before, UK faces the challenges of more spending and less revenue. The primary and the most direct benefit of this project is to significantly reduce the cost for prevalence profiling of multiple NCD, having great potential to make the current workflow of public health authorities much cheaper and more efficiently.
- Ordinary residents: The spatially fine-grained prevalence profile of multiple NCD helps those living high-prevalence regions draw more attention from both the government and society. This may benefit them from obtaining more facilities (e.g., green space and exercise facilities), allocated medical resources (e.g., more deployed GP), charity services (education on healthy lifestyles), and social care. Also, the findings from this project will help themselves reflect on the environment factors and their lifestyles related to the high prevalence rate of certain diseases.
- Health data science research community: this project aims to disseminate the research outcome through publications in the best conferences and journals, and we will develop prototypes and websites which are available to the researchers of data science and digital health. Also, we will build up collaborations with world-leading research groups through academic visits and organization of multiple workshops. In addition, the outcomes of this project have the potential to be adopted worldwide with appropriate customizations and adjustments, which will strengthen the UK's international leadership position in the research community of health data science
Outputs
- At least two published papers
- Conference attendance- protype demonstration/ tutorials
- 4 workshops to be hosted at Coventry University